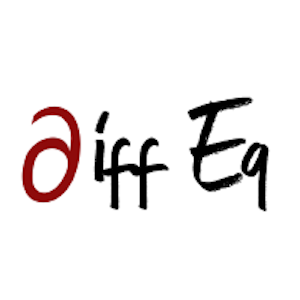
One-Shot Transfer Learning for Nonlinear Differential Equations with Neural Networks
The ability to rapidly adapt neural networks for solving various differential equations holds immense potential. Achieving "one-shot transfer learning" would pave the way for foundational models applicable to entire families of differential equations, encompassing both ordinary (ODEs) and partial differential equations (PDEs). Such models could efficiently handle diverse initial conditions, forcing functions, and other parameters, offering a universally reusable solution framework.
Background and Prior Work:
Our research has made significant strides in this direction. We previously demonstrated one-shot transfer learning for linear equations [1,2]. Subsequently, we built upon this success by employing perturbation methods to achieve iterative one-shot transfer learning for simple polynomial nonlinearities in differential equations [3].
Project Goals:
This project aims to extend our prior work by tackling non-polynomial nonlinearities in differential equations. While our prior work utilized the homotopy perturbation method, its limited convergence regions pose a challenge. Here, we propose exploring alternative expansion techniques, such as Pade approximations [4], as a means to effectively handle a broader range of nonlinearities.
Methodology:
- Exploration of Expansion Techniques: We will delve into Pade approximations and potentially other expansion methods suitable for representing diverse nonlinearities in differential equations.
- Model Development: We will integrate the chosen expansion technique into a neural network architecture, enabling the model to learn the solution structure for various non-polynomial nonlinearities.
- Benchmarking and Validation: The model's performance will be evaluated across a diverse set of ODEs and PDEs.
- Real-World Application: We will select a specific real-world application involving non-polynomial nonlinearities and demonstrate the effectiveness of the developed model in solving the corresponding differential equations.
References:
One-shot transfer learning of physics-informed neural networks
Generalized One-Shot Transfer Learning of Linear Ordinary and Partial Differential Equations
- Algebraic approximants and the numerical solution of parabolic equations
Future Directions in Stiffness Modeling: Expanding Multi-Head PINNs
Ordinary differential equations (ODEs) are fundamental in modeling a vast range of physical, biological, and engineering systems. However, solving these equations, particularly for stiff systems, remains a significant computational challenge. Stiffness arises when solutions evolve on vastly different timescales, requiring specialized numerical methods to capture rapid transients and slow dynamics simultaneously. Traditional solvers like Runge-Kutta methods often struggle with efficiency and stability, necessitating extremely small time steps for stiff systems. This inefficiency is amplified when exploring varying initial conditions or force functions within stiff regimes.
In this context, Physics-Informed Neural Networks (PINNs) offer a promising alternative. By integrating governing equations into neural network structures via automatic differentiation, PINNs can approximate solutions directly without traditional mesh-based discretization. Building on this foundation, this work introduces a novel multi-head PINN architecture and leverages transfer learning to address the unique challenges of stiffness. These methods aim to improve computational efficiency and broaden the applicability of PINNs across diverse stiffness regimes.
Previous Work
In our prior work [1], we proposed a novel approach to solving stiff ODEs using Physics-Informed Neural Networks (PINNs) with a multi-head architecture and transfer learning. By integrating governing equations directly into neural networks through automatic differentiation, PINNs provide an alternative to traditional numerical solvers.
Our method introduced a multi-head architecture, where each “head” specializes in a specific stiffness regime. The network was first trained on non-stiff regimes, then fine-tuned for stiff systems using transfer learning to leverage pre-trained weights. This strategy significantly reduced computational costs compared to methods like RK45 and Radau, particularly when exploring varying initial conditions or force functions.
We validated the approach on benchmark linear and nonlinear ODEs with varying stiffness ratios, demonstrating improvements in accuracy and execution time over vanilla PINNs and traditional solvers.
Future Work
Building on the success of this project, we aim to extend the applicability of the proposed approach in the following directions:
- Extension to Stiff PDEs: Expand the use of the multi-head architecture and transfer learning to partial differential equations (PDEs) with stiff dynamics. This includes addressing complex problems like the one-dimensional advection-reaction system, a benchmark in atmospheric modeling [2, 3], and extending to systems relevant in fluid dynamics and materials science.
- Broadening Stiffness Regime Coverage: Investigate the effectiveness of the multi-head architecture across diverse stiffness types, such as boundary layer stiffness, oscillatory stiffness, and thermal runaway stiffness. This work aims to generalize the methodology for applicability to various domains.
- Applications in Astronomy and Physics: Explore the use of this framework for astrophysical simulations, such as modeling stellar interiors, planetary atmospheres, or accretion disk dynamics, where stiffness arises from coupled thermodynamic and radiative processes. Similarly, in physics, apply the method to problems like plasma dynamics or high-energy particle interactions, where disparate timescales and sharp gradients are prevalent.
- Other High-Impact Domains: Extend the approach to industrial applications, including chemical reaction networks, biological systems, and climate modeling, which often involve stiff systems and require efficient, accurate solvers.
References
- Emilien Sellier and Pavlos Protopapas, submitted to AISTATS
- Physics-informed neural networks for stiff partial differential equations with transfer learning.
- Brasseur, G. P., & Jacob, D. J. (2017). Atmospheric chemistry and global change. Oxford University Press.