Educational Activities and Philosophy
In this page, I outline my educational activities and philosophy. These encompass not only the formal courses I teach but workshops and less formal educational experiences that lie outside traditional coursework.Courses
Many of the members of the lab are in some way or another involved in the teaching of the following courses.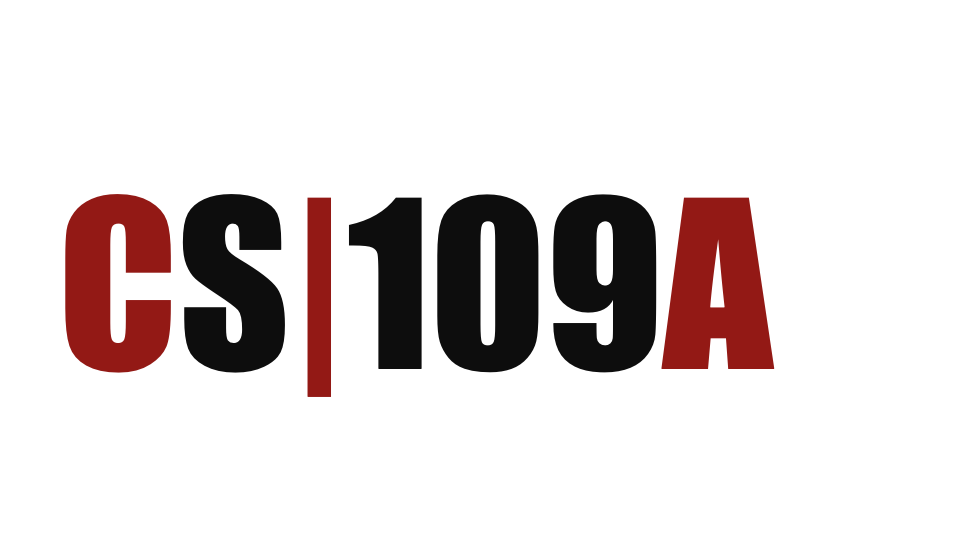
CS109A
Introduction to Data Science.
This is an introduction to data science being taught at Harvard every fall. The course
covers data scrapping, data visualization, basic machine learning such as decision trees and regression models for prediction.
and statistical inference in regressions.
1. data collection - data wrangling, cleaning, and sampling to get a suitable
data set;
2. data management - accessing data quickly and reliably;
3. exploratory data analysis – generating hypotheses and building intuition;
4. prediction or statistical learning; and
5. communication – summarizing results through visualization, stories, and
interpretable summaries.
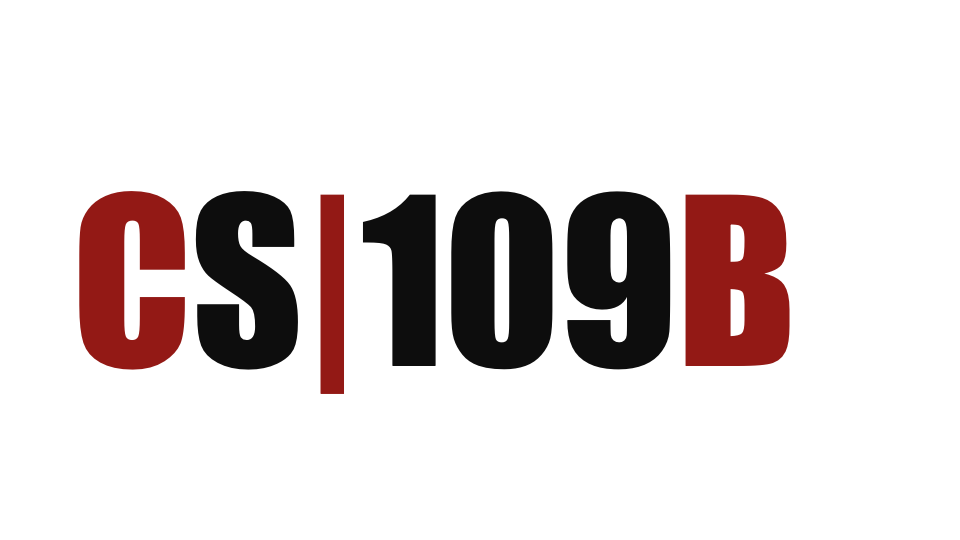
CS109B
Advanced Topics in Data Science.
Advanced Topics in Data Science (CS109b) is the second half of a one-year introduction to data science.
Building upon the material in Introduction to Data Science (CS109A), the course introduces advanced methods for data wrangling, data visualization, statistical modeling, and prediction. Topics include big data, multiple deep learning architectures such as CNNs, RNNs, language models, transformers, autoencoders,
and generative models as well as Bayesian modeling, sampling methods, and unsupervised learning.
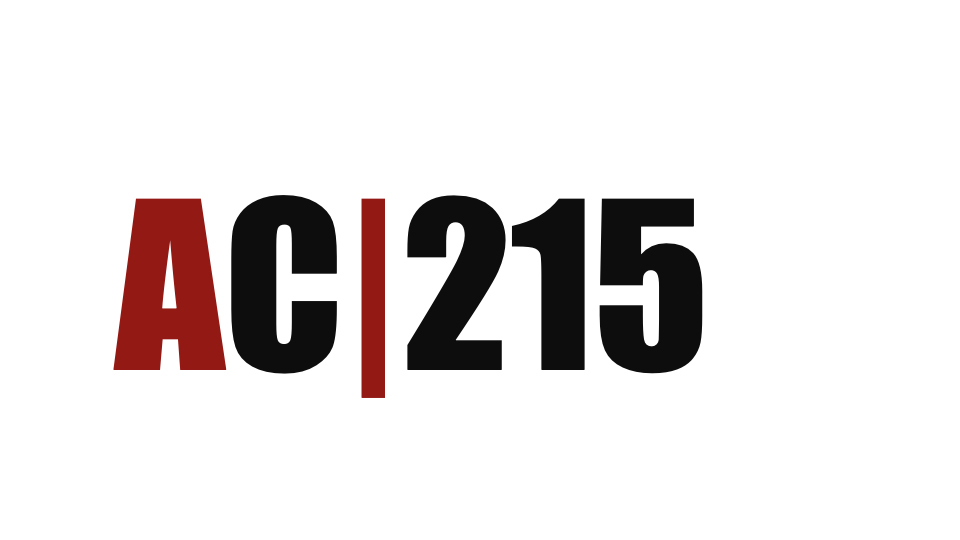
AC215
In today’s AI-driven landscape, building a deep learning model is just the beginning; the real challenge lies in making it scalable, maintainable, and deployment-ready. AC215: Productionizing AI (Machine Learning Operations) focuses on the entire ML operations workflow, particularly for Large Language Models (LLMs). This course covers essentials like containerization, cloud functions, data pipelines, and advanced training techniques, with a special emphasis on LLM applications. You’ll learn to use LLM APIs, fine-tune models for specific tasks, and build scalable applications, gaining the skills to deploy AI in real-world scenarios effectively.
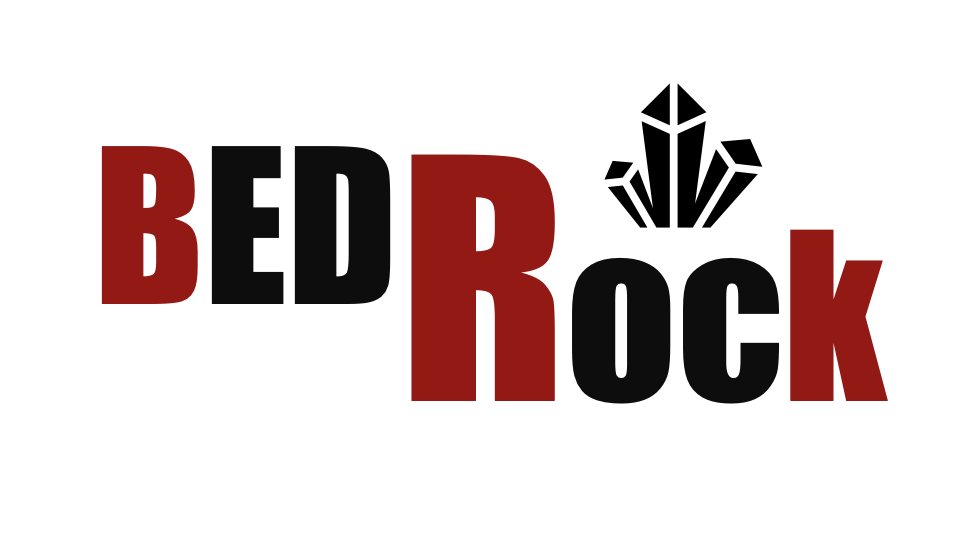
Bedrock Data Science
The objective of this course is to provide fundamental understanding of math, statistics & programming required to undertake a course in machine learning, data science or AI. You will start with the basics of python, statistics, linear algebra, and calculus. By the end of the course, you will have the tools and know the concepts needed to successfully undertake a rigorous course in machine learning.
Course Topics:
- Basic Python: Data types, data structures, functions
- Advanced Python: Python Classes
- Probability & Statistics
- Linear Algebra & Calculus
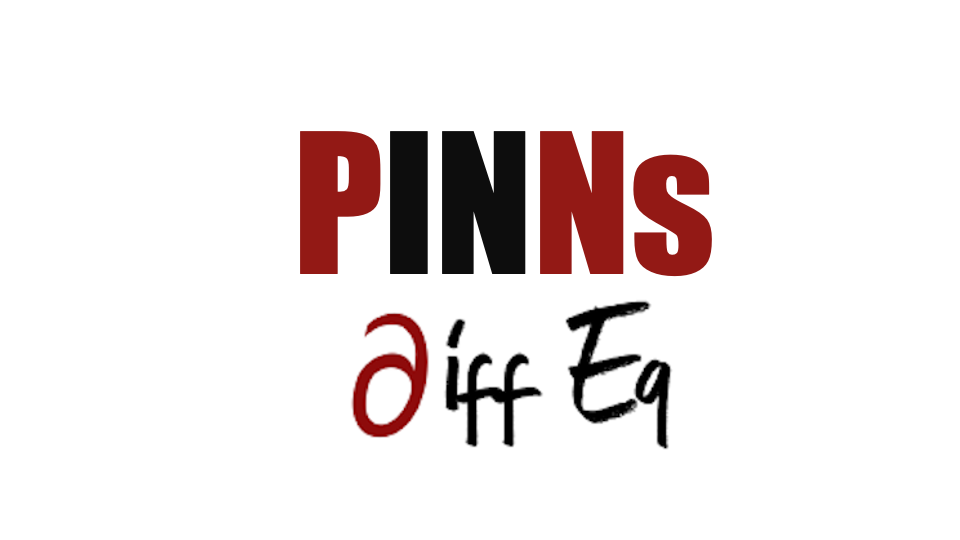
The PINNS course
In this course, we will first review differential equations and traditional numerical methods to solve them. We will then delve into neural network approaches for solving differential equations, initial and boundary condition problems, optimization methods, sampling techniques, error characterization, and transfer learning. At the end of the course, students will be able to understand the challenges and advantages of PINNs, know different approaches and compare them, and be able to implement and use existing libraries to solve linear and non-linear ODE, a system of ODEs, a system of linear and not linear-PDEs.
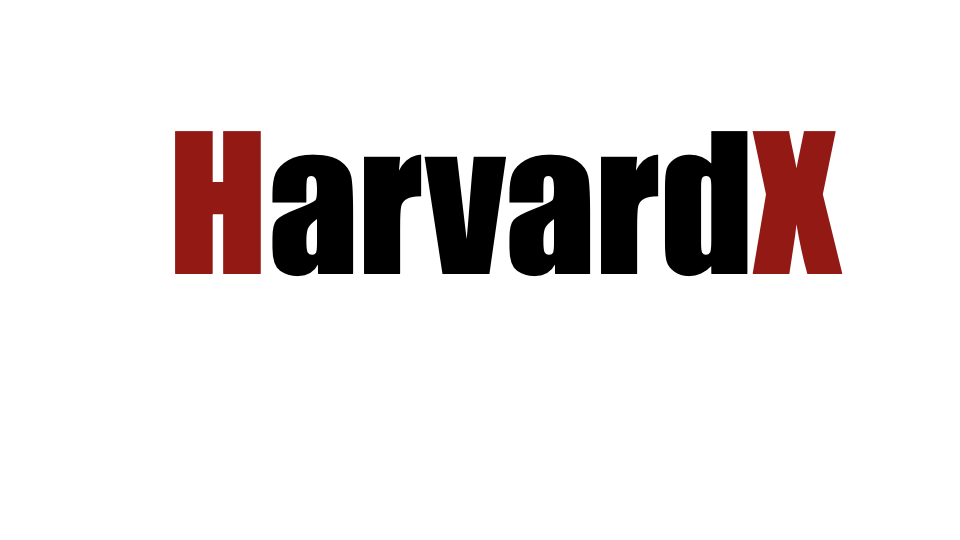
Introduction to Data Science with Python
This course guides learners through essential data science techniques using Python,
covering regression, classification, and libraries like sklearn
and Pandas
.
Key ML concepts such as overfitting, regularization, and model evaluation are introduced,
providing a strong foundation in Python for advanced study in Machine Learning and AI.
Machine Learning and AI with Python
Focusing on decision-making through machine learning, this course introduces decision trees, progressing to bagging, random forests, and gradient boosting. Real-world cases help learners practice prediction, refine models, and address issues like overfitting and bias, preparing them for complex decision-making using Python.
Know more hereCourses
Here I outline various teaching activities that I have been involved but do not fall under structured courses.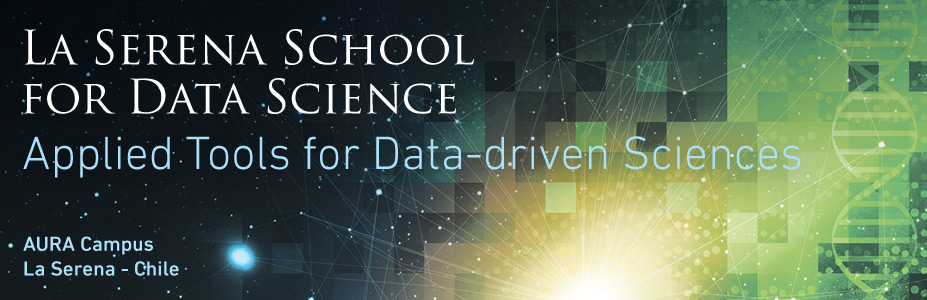
La Serena School for Data Science (LSSDS), La Serena, Chile
La Serena School for Data Science (LSSDS), La Serena, Chile.
LSSDS.
I have been a regular faculty member at the La Serena School for
Data Science (LSSDS), an intensive 10-day program in La Serena, Chile,
focused on big data analysis, especially for applications in astronomy.
Since 2013, LSSDS has offered training in machine learning,
cloud computing, and statistical tools, with interdisciplinary lectures
and hands-on team projects using real data. This program is designed for
advanced undergraduates and early graduate students from the U.S., Chile,
Ecuador, and other Latin American countries. During my participation,
I have served as the lead faculty for machine learning and mentored
students on their projects.
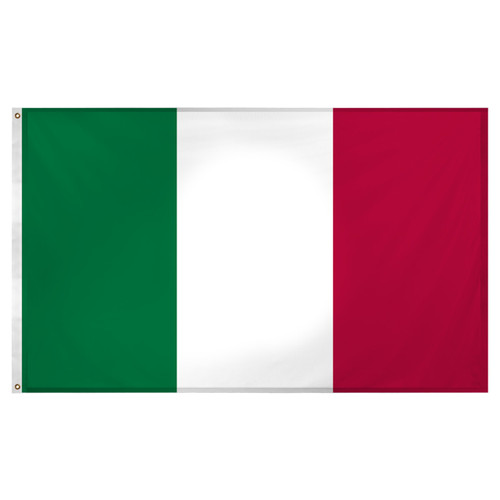
HPE Group, AI Workshop, Modena, Italy
HPE Group, AI Workshop, Modena, Italy.
A specialized course on sequential data and language models using deep
neural networks was designed and delivered to a cohort of 5 engineers at
HPE Group, a leading provider of engineering solutions based in
Modena, Italy. This synchronous online course focused on advanced topics
such as recurrent neural networks, attention mechanisms, and transformer
architecture. Delivered over nine weeks, the course included nine lectures
and nine hands-on lab sessions, providing participants with a comprehensive
understanding of these cutting-edge technologies.
Rwanda Data Science School
Rwanda Data Science School.
The Rwanda Data Science School, held in Rwanda during summer 2019
and transitioned online in 2020-2021, was an intensive three-week program
introducing fundamental data science concepts. As one of two lead faculty members,
I helped develop and deliver curriculum to approximately 50 participants.
The program covered data scraping, basic machine learning models, and data visualization
through hands-on learning experiences. With three lectures per week, participants
gained practical skills in data analysis and visualization, preparing them for
real-world applications in data science.